Large language models (LMs) are capable of in-context learning from a few demonstrations (example-label pairs) to solve new tasks during inference. Despite the intuitive importance of high-quality demonstrations, previous work has observed that, in some settings, ICL performance is minimally affected by irrelevant labels (Min et al., 2022). We hypothesize that LMs perform ICL with irrelevant labels via two sequential processes: an inference function that solves the task, followed by a verbalization function that maps the inferred answer to the label space. Importantly, we hypothesize that the inference function is invariant to remappings of the label space (e.g., “true”/“false” to “cat”/“dog”), enabling LMs to share the same inference function across settings with different label words. We empirically validate this hypothesis with controlled layer-wise interchange intervention experiments. Our findings confirm the hypotheses on multiple datasets and tasks (natural language inference, sentiment analysis, and topic classification) and further suggest that the two functions can be localized in specific layers across various open-sourced models, including GEMMA-7B, MISTRAL-7B-V0.3, GEMMA-2-27B, and LLAMA-3.1-70B.
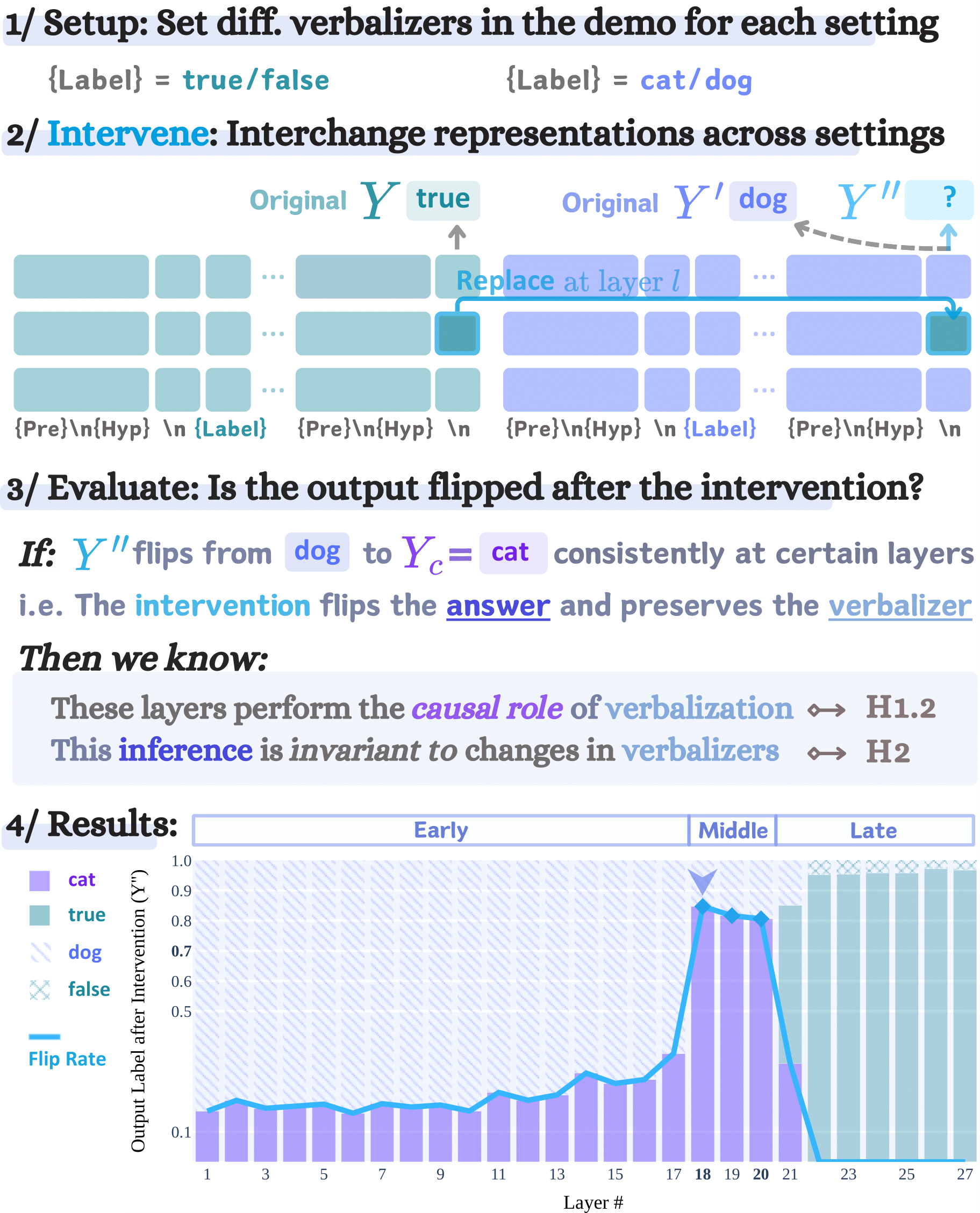